Persistent activity is problematic as a working memory (WM) mechanism. When WMs are in a persistent active state, they are easy to disrupt by distractions, additional inputs etc. Using modeling, Freedman and crew show that during simple WM maintenance, neural activity is transient, not persistent. This makes sense because the less often memories are in an active state, the better they are protected from disruption. However, when working memories must be manipulated and changed, the activity is more persistent. This makes sense because you want memories to be in a labile, changeable, state when you are manipulating them.
Circuit mechanisms for the maintenance and manipulation of information in working memory
Nicolas Y. Masse, Guangyu R. Yang, H. Francis Song, Xiao-Jing Wang & David J. Freedman
For further reading see:
Lundqvist, M., Herman, P., and Miller, E.K. (2018) Working Memory: Delay Activity, Yes! Persistent Activity? Maybe not. Journal of Neuroscience, 8 August 2018, 38 (32) 7013-7019; DOI: https://doi.org/10.1523/JNEUROSCI.2485-17.2018. View PDF
Miller, E.K., Lundqvist, L., and Bastos, A.M. (2018) Working Memory 2.0 Neuron, DOI:https://doi.org/10.1016/j.neuron.2018.09.023 View PDF
Circuit mechanisms for the maintenance and manipulation of information in working memory
Nicolas Y. Masse, Guangyu R. Yang, H. Francis Song, Xiao-Jing Wang & David J. Freedman
For further reading see:
Lundqvist, M., Herman, P., and Miller, E.K. (2018) Working Memory: Delay Activity, Yes! Persistent Activity? Maybe not. Journal of Neuroscience, 8 August 2018, 38 (32) 7013-7019; DOI: https://doi.org/10.1523/JNEUROSCI.2485-17.2018. View PDF
Miller, E.K., Lundqvist, L., and Bastos, A.M. (2018) Working Memory 2.0 Neuron, DOI:https://doi.org/10.1016/j.neuron.2018.09.023 View PDF
Widge, A.S., Zorowitz, S., Basu, I. Paulk, A., Cash, S., Eskandar, E., Deckersbach, T., Miller, E.K., and Dougherty, D. (2019) Deep brain stimulation of the internal capsule
Summary:
Deep brain stimulation (DBS) is a circuit-oriented treatment for mental disorders. Unfortunately, even well-conducted psychiatric DBS clinical trials have yielded inconsistent symptom relief, in part because DBS’ cognitive and physiologic mechanism(s) of action are unclear. One clue to those mechanisms may lie in DBS’ efficacy in both major depression (MDD) and obsessive-compulsive disorder (OCD) by stimulation of a single brain site, the ventral internal capsule/ventral striatum (VCVS). MDD and OCD both involve deficits in cognitive control. Cognitive control depends on prefrontal cortex (PFC) regions that project into the VCVS. We show that VCVS DBS’ effect is explained in part by
More evidence that cognitive control stems from low-frequency rhythms in deep-layer cortex inhibiting superficial layer cortex.
Johnston, K., Ma, L., Schaeffer, L., & Everling, S. (2019). Alpha Oscillations Modulate Preparatory Activity in Marmoset Area 8Ad. Journal of Neuroscience, 39(10), 1855-1866.
For further reading see:
Miller, E.K., Lundqvist, L., and Bastos, A.M. (2018) Working Memory 2.0 Neuron, DOI:https://doi.org/10.1016/j.neuron.2018.09.023 View PDF
Bastos, A.M., Loonis, R., Kornblith, S., Lundqvist, M., and Miller, E.K. (2018) Laminar recordings in frontal cortex suggest distinct layers for maintenance and control of working memory. Proceedings of the National Academy of Sciences. View PDF
Lundqvist, M., Herman, P. Warden, M.R., Brincat, S.L., and Miller, E.K. (2018) Gamma and beta bursts during working memory read-out suggest roles in its volitional control. Nature Communications. 9, 394 View PDF
Lundqvist, M., Rose, J., Herman, P, Brincat, S.L, Buschman, T.J., and Miller, E.K. (2016) Gamma and beta bursts underlie working memory. Neuron, published online March 17, 2016. View PDF »
This paper provides further evidence for different roles for beta vs gamma oscillations
Riddle, J., Hwang, K., Cellier, D., Dhanani, S., & D’Esposito, M. (2019). Causal Evidence for the Role of Neuronal Oscillations in Top–Down and Bottom–Up Attention. Journal of cognitive neuroscience, 1-12.
For further info see:
Buschman, T.J. and Miller, E.K. (2007) Top-down versus bottom-up control of attention in the prefrontal and posterior parietal cortices. Science. 315: 1860-1862 View PDF
Lundqvist, M., Rose, J., Herman, P, Brincat, S.L, Buschman, T.J., and Miller, E.K. (2016) Gamma and beta bursts underlie working memory. Neuron, published online March 17, 2016. View PDF »
Lundqvist, M., Herman, P. Warden, M.R., Brincat, S.L., and Miller, E.K. (2018) Gamma and beta bursts during working memory read-out suggest roles in its volitional control. Nature Communications. 9, 394 View PDF
Here’s a review:
Miller, E.K., Lundqvist, L., and Bastos, A.M. (2018) Working Memory 2.0 Neuron, DOI:https://doi.org/10.1016/j.neuron.2018.09.023 View PDF
Widge, A.S., Boggess, M., Rockhill, A.P., Mullen, A., Sheopory, S., Loonis, R. Freeman, D.K., and Miller, E.K. (2018) Altering alpha-frequency brain oscillations with rapid analog feedback-driven neurostimulation. PLOS ONE
Abstract
Oscillations of the brain’s local field potential (LFP) may coordinate neural ensembles and brain networks. It has been difficult to causally test this model or to translate its implications into treatments because there are few reliable ways to alter LFP oscillations. We developed a closed-loop analog circuit to enhance brain oscillations by feeding them back into cortex through phase-locked transcranial electrical stimulation. We tested the system in a rhesus macaque with chronically implanted electrode arrays, targeting 8–15 Hz (alpha) oscillations. Ten seconds of stimulation increased alpha oscillatory power for up to 1 second after stimulation offset. In contrast, open-loop stimulation decreased alpha power. There was no effect in the neighboring 15–30 Hz (beta) LFP rhythm or on a neighboring array that did not participate in closed-loop feedback. Analog closed-loop neurostimulation might thus be a useful strategy for altering brain oscillations, both for basic research and the treatment of neuro-psychiatric disease
An article in Scientific American discusses the debate about whether brain waves have a function or whether they are an epiphenomenon:
Do Brain Waves Conduct Neural Activity Like a Symphony? There are so many things wrong with this debate.
We know so little about how the brain functions. To dismiss observable signals as an epiphenomenon assumes a level of knowledge that no one has. It is merely a defense of the status quo paradigm, straight out of Thomas Kuhn. The attitude boils down to “they don’t play a role because we kind of already know how the brain works”. No, you don’t. No one does.
What do neurons do?: They spike when their membrane potential reaches the spiking threshold. What do oscillations do? They move the membrane potential towards and away from the spiking threshold. You start with the assumption that oscillations matter, not that they don’t. The latter is just a defense of what you think you already know. That attitude holds back progress.
The article says that “critics point out that oscillations arise everywhere one looks in nature” so therefore brain oscillations are not functional. To my mind, that is an argument *for* a functional role. Evolution builds on what is already available.
Critics also say that there is not a lot of evidence for a link between oscillations and mental states. Yes, there is. There are more and more papers published each week. There might be more evidence for spiking but that is just because spiking has been studied longer. The same type of correlational evidence is there for both spikes and oscillations. As for the lack of a causal role between oscillations and brain function, the evidence for a causal role between spiking and function is equally flimsy. Spiking is not the gold standard just because it is the first and easiest thing we could measure when we only had single-channel amplifiers and slow or no computers.
Skepticism is a good thing but where is the skepticism about the notion that spikes do it all? For example, consider the following quote from the article: “evidence amassed so far is not based on rigorous tests looking for a cause-and-effect relationship between gamma waves and specific neural processes.” The same statement is equally true for cause-and-effect between spikes and function. Somehow that gets a free pass? The oscillation naysayers accept their spikes-only model without question but set a high bar for others. I refer you back to Thomas Kuhn.
Spikes vs oscillations is not an either/or thing. They both work together. Indeed, it is hard to imagine how one would decouple them. The bottom line is that both spikes and oscillations are both signals. No one knows enough about how the brain works to dismiss the measuring of a signal. Since when is more data a bad thing?
Widge AS, Boggess M, Rockhill AP, Mullen A, Sheopory S, Loonis R, Freeman DK, Miller EK (in press) Altering alpha-frequency brain oscillations with rapid analog feedback-driven neurostimulation. PLOS ONE
Stayed tuned for the exciting details.
Check out this National Public Radio piece on the debate about our new model of working memory:
Neuroscientists Debate A Simple Question: How Does The Brain Store A Phone Number? I have a few follow-up points.
1. In the piece, Christos Constantinidis says: “The problem with the theory is that so far there has been no experimental evidence linking this critical variable with behavior,”
This is false. We have a paper entirely devoted to experimental evidence linking the theory to behavior:
Lundqvist, M., Herman, P. Warden, M.R., Brincat, S.L., and Miller, E.K. (2018) Gamma and beta bursts during working memory read-out suggest roles in its volitional control. Nature Communications. 9, 394 View PDF
2. Christos also says: Miller’s contention that working memory is linked to long-term memory seems at odds with doctors’ experience with patients whose brains have been injured.
This totally misses the point. Working memory can exploit some of the same mechanisms as long-term memory (synaptic weight changes) while at the same time rely on different brain areas. The fact that you can have brain damage that disrupts working memory without disrupting long-term memory is completely irrelevant.
3. Most importantly, the people who support the old model of persistent activity have not done the crucial test. All the evidence for the old model of persistent activity averages activity across trials. You cannot do this. Averaging creates an illusion of persistence. You must examine activity on single trials. Unless you do that, you are not addressing the issue.
If you want to read about our new working memory, check out this paper:
Miller, E.K., Lundqvist, L., and Bastos, A.M. (2018) Working Memory 2.0 Neuron, DOI:https://doi.org/10.1016/j.neuron.2018.09.023 View PDF
Our new paper describing a new model of working memory. Actually, not so much a new model as an update to the classic model. The classic model posited that we hold thoughts “in mind” (i.e., in working memory) via the persistent spiking of neurons. That is not wrong. It is right to a certain level of approximation. There is little doubt that spikes help maintain working memories. However, a closer examination revealed that there is much more going on than persistent spiking.
It is important to keep in mind (pun intended) that virtually all evidence for persistent spiking comes from experiments that averaged neural activity across trials. The assumption was that averaging boosts signal and decreases noise (“noise” meaning changes in activity from trial to trial). But what if that noise was not noise but real neural dynamics? We don’t always think about the same thing in the same way. Averaging assumes we do.
With this in mind, we and others have been leveraging multiple-electrode recording to examine neural activity in “real time” (on individual trials). This has revealed that working memory-related spiking occurs in sparse, coordinated bursts of activity. It also revealed oscillatory dynamics between brain waves in two frequency bands, beta and gamma. Gamma seems to act as a carrier wave that maintains the contents of working memory. Beta seems carry the top-down control signals that allow us to exert volitional control over working memory.
Miller, E.K., Lundqvist, M., and Bastos, A.M. Working Memory 2.0 Neuron DOI:https://doi.org/10.1016/j.neuron.2018.09.023 (Download a *free* copy for the next 50 days)
Here’s the abstract:
Working memory is the fundamental function by which we break free from reflexive input-output reactions to gain control over our own thoughts. It has two types of mechanisms: online maintenance of information and its volitional or executive control. Classic models proposed persistent spiking for maintenance but have not explicitly addressed executive control. We review recent theoretical and empirical studies that suggest updates and additions to the classic model. Synaptic weight changes between sparse bursts of spiking strengthen working memory maintenance. Executive control acts via interplay between network oscillations in gamma (30–100 Hz) in superficial cortical layers (layers 2 and 3) and alpha and beta (10–30 Hz) in deep cortical layers (layers 5 and 6). Deep-layer alpha and beta are associated with top-down information and inhibition. It regulates the flow of bottom-up sensory information associated with superficial layer gamma. We propose that interactions between different rhythms in distinct cortical layers underlie working memory maintenance and its volitional control.
V1-V4 gamma coherence before stimulus change predicts reaction time to detect the change while deviations from the phase relation increases reaction times. Nice.
Gamma Synchronization between V1 and V4 Improves Behavioral Performance
Gustavo Rohenkohl, Conrado Arturo Bosman, Pascal Fries
A trial by trial analysis showed that beta bursts, as opposed to power averaged across trials, is a good predictor of variations in motor behavior.
Torrecillos, F., Tinkhauser, G., Fischer, P., Green, A. L., Aziz, T. Z., Foltynie, T., … & Tan, H. (2018). Modulation of beta bursts in the subthalamic nucleus predicts motor performance. Journal of Neuroscience, 38(41), 8905-8917.
Rodu, J., Klein, N., Brincat, S. L., Miller, E. K., & Kass, R. E. (2018). Detecting Multivariate Cross-Correlation Between Brain Regions. Journal of neurophysiology.
Abstract
The problem of identifying functional connectivity from multiple time series data recorded in each of two or more brain areas arises in many neuroscientific investigations. For a single stationary time series in each of two brain areas statistical tools such as cross-correlation and Granger causality may be applied. On the other hand, to examine multivariate interactions at a single time point, canonical correlation, which finds the linear combinations of signals that maximize the correlation, may be used. We report here a new method that produces interpretations much like these standard techniques and, in addition, 1) extends the idea of canonical correlation to 3-way arrays (with dimensionality number of signals by number of time points by number of trials), 2) allows for nonstationarity, 3) also allows for nonlinearity, 4) scales well as the number of signals increases, and 5) captures predictive relationships, as is done with Granger causality. We demonstrate the effectiveness of the method through simulation studies and illustrate by analyzing local field potentials recorded from a behaving primate.
When MIT neuroscientist Earl Miller was in graduate school at Princeton, he was inspired by the lectures of George A. Miller, an influential psychologist who helped to spark the young student’s interest in working memory. Now, as the newly named 2019 recipient of the George A. Miller Prize in Cognitive Neuroscience, Earl Miller is set to deliver a lecture honoring his teacher at the annual meeting of the Cognitive Neuroscience Society in San Francisco in March.
Read more here.
Nice review from Miler Lab alumnus Joni Wallis arguing for the importance of single-trial analyses. Variability across trials may not be noise, it may be cognition. Joni argues that ensembles, not single neurons, are the fundamental unit in the brain. One needs to record from many neurons simultaneously to understand cognitive processes.
Wallis, J. D. (2018). Decoding Cognitive Processes from Neural Ensembles. Trends in Cognitive Sciences.
This review highlights work showing that spectrally distributed oscillations and their coupling have functional relevance for sensorimotor processing.
Palva, S., & Palva, J. M. (2018). Roles of brain criticality and multiscale oscillations in temporal predictions for sensorimotor processing. Trends in Neurosciences, 41(10), 729-743.
Nice FMRI study showing that working memory delay activity is primarily in the superficial, feedforward, cortical layers while behavioral response-related activity is primarily in deep, feedback layers.
Layer-dependent activity in human prefrontal cortex during working memory
Emily S. Finn, Laurentius Huber, David C. Jangraw, Peter A. Bandettini
doi: https://doi.org/10.1101/425249
This is very consistent with our recent work:
Bastos, A.M., Loonis, R., Kornblith, S., Lundqvist, M., and Miller, E.K. (2018) Laminar recordings in frontal cortex suggest distinct layers for maintenance and control of working memory. Proceedings of the National Academy of Sciences. View PDF
Holmes, C.D., Papadimitriou, C., Snyder, L.H.(2018) Dissociation of LFP Power and Tuning in the Frontal Cortex during Memory Journal of Neuroscience
Nice paper. Well done. But with a caveat. The authors show that absolute power is dissociated from neural tuning in spiking activity. From this, they conclude that “oscillatory activity by itself is likely not a substrate of memory” and “may be an epiphenomenon of a rate code in the circuit, rather than a direct substrate”.
Not quite. No one is claiming that absolute power alone carries specific information. Rather, it is *patterns of coherence* that carry information (e.g., Buschman et al., 2012; Salazar et al 2012; Antzoulatos and Miller, 2014). If so, there is no reason to think that information would be carried by absolute power. For example, two different patterns of coherence for two different items could have equal global power because it is the pattern, not the global power, that matters. In fact, we and others have shown that coherence and power can be dissociated (Buschman et al., 2012). Using absolute power as a proxy to argue against a functional role for oscillations is a “straw man” argument. It tests a hypothesis that does not reflect the state-of-the-art of thinking on this matter.
Further reading:
Antzoulatos, E.G. and Miller, E.K. (2014) Increases in functional connectivity between the prefrontal cortex and striatum during category learning. Neuron, 83:216-225. View PDF »
Buschman, T.J., Denovellis, E.L., Diogo, C., Bullock, D. and Miller, E.K. (2012) Synchronous oscillatory neural ensembles for rules in the prefrontal cortex. Neuron. 76: 838-846. View PDF »
Salazar, R.F., Dotson, N.M., Bressler, S.L., and Gray, C.M. (2012). Content-Specific Fronto-Parietal Synchronization During Visual Working Memory. Science 1224000
Another point: The reason they see “tuning” for contra vs ipsilateral targets in power is not because of stimulus tuning per se, it is because the right vs left visual hemifields are somewhat independent. See:
Buschman,T.J., Siegel, M., Roy, J.E. and Miller, E.K. (2011) Neural substrates of cognitive capacity limitations. Proceedings of the National Academy of Sciences. 108(27):11252-5. View PDF »
Kornblith, S., Buschman, T.J., and Miller, E.K. (2015) Stimulus load and oscillatory activity in higher cortex. Cerebral Cortex. Published online August 18, 2015 doi: 10.1093/cercor/bhv182. View PDF »
On the role of cortex-basal ganglia interactions for category learning: A neuro-computational approach
Francesc Villagrasa, Javier Baladron, Julien Vitay, Henning Schroll, Evan G. Antzoulatos, Earl K. Miller and Fred H. Hamker
Journal of Neuroscience 18 September 2018, 0874-18; DOI: https://doi.org/10.1523/JNEUROSCI.0874-18.2018
Abstract
In addition to the prefrontal cortex (PFC), the basal ganglia (BG) have been increasingly often reported to play a fundamental role in category learning, but the systems-level circuits of how both interact remain to be explored. We developed a novel neuro-computational model of category learning that particularly addresses the BG-PFC interplay. We propose that the BG bias PFC activity by removing the inhibition of cortico-thalamo-cortical loop and thereby provide a teaching signal to guide the acquisition of category representations in the cortico-cortical associations to the PFC. Our model replicates key behavioral and physiological data of macaque monkey learning a prototype distortion task from Antzoulatos and Miller (2011). Our simulations allowed us to gain a deeper insight into the observed drop of category selectivity in striatal neurons seen in the experimental data and in the model. The simulation results and a new analysis of the experimental data, based on the model’s predictions, show that the drop in category selectivity of the striatum emerges as the variability of responses in the striatum rises when confronting the BG with an increasingly larger number of stimuli to be classified. The neuro-computational model therefore provides new testable insights of systems-level brain circuits involved in category learning which may also be generalized to better understand other cortico-basal ganglia-cortical loops
Nice study showing that anterior parts of the prefrontal cortex and more plastic than posterior parts.
Anterior-posterior gradient of plasticity in primate prefrontal cortex
Mitchell R. Riley, Xue-Lian Qi, Xin Zhou & Christos Constantinidis
Nature Communications volume 9, Article number: 3790 (2018)
Zanos et al show that beta oscillations play a role in short-term synaptic plasticity in primate neocortex that may explain the role of oscillations in attention, learning, and cortical reorganization.
Zanos, S., Rembado, I., Chen, D., & Fetz, E. E. (2018). Phase-locked stimulation during cortical beta oscillations produces bidirectional synaptic plasticity in awake monkeys. Current Biology.
See discussion of this paper by Womelsdorf and Hoffman:
Latent Connectivity: Neuronal Oscillations Can Be Leveraged for Transient Plasticity
Bouchacourt and Buschman describe a two-layer model of working memory. A sensory layer feeds into an unstructured layer of neurons with random connections (i.e., “mixed-selectivity” type neurons). It is flexible but interference between representations results in a capacity limit. Sounds like working memory to me.
Bouchacourt, F., & Buschman, T. J. (2018). A Flexible Model of Working Memory. bioRxiv, 407700.
More about mixed-selectivity:
Fusi, S., Miller, E.K., and Rigotti, M. (2016) Why neurons mix: High dimensionality for higher cognition. Current Opinion in Neurobiology. 37:66-74 doi:10.1016/j.conb.2016.01.010. View PDF »
Rigotti, M., Barak, O., Warden, M.R., Wang, X., Daw, N.D., Miller, E.K., & Fusi, S. (2013) The importance of mixed selectivity in complex cognitive tasks. Nature, 497, 585-590, doi:10.1038/nature12160. View PDF »
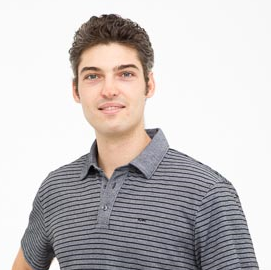
Congrats to Miller Lab postdoc Andre Bastos for being awarded a prestigious K99 Award from the National Institutes of Health.
Nice summary of phase coding models of working memory by Hakim and Vogel, including a recent paper by Bahramisharif et al.
Hakim, N., & Vogel, E. K. (2018). Phase-coding memories in mind. PLoS biology, 16(8), e3000012.
Bahramisharif, A., Jensen, O., Jacobs, J., & Lisman, J. (2018). Serial representation of items during working memory maintenance at letter-selective cortical sites. PLoS biology, 16(8), e2003805.
Wasmuht, D. F., Spaak, E., Buschman, T. J., Miller, E. K., & Stokes, M. G. (2018). Intrinsic neuronal dynamics predict distinct functional roles during working memory. Nature Communications.
Abstract:
Working memory (WM) is characterized by the ability to maintain stable representations over time; however, neural activity associated with WM maintenance can be highly dynamic. We explore whether complex population coding dynamics during WM relate to the intrinsic temporal properties of single neurons in lateral prefrontal cortex (lPFC), the frontal eye fields (FEF), and lateral intraparietal cortex (LIP) of two monkeys (Macaca mulatta). We find that cells with short timescales carry memory information relatively early during memory encoding in lPFC; whereas long-timescale cells play a greater role later during processing, dominating coding in the delay period. We also observe a link between functional connectivity at rest and the intrinsic timescale in FEF and LIP. Our results indicate that individual differences in the temporal processing capacity predict complex neuronal dynamics during WM, ranging from rapid dynamic encoding of stimuli to slower, but stable, maintenance of mnemonic information.
Two new, exciting papers in Neuron that “put the last nail(s) in the coffin of sustained attention.” They present compelling evidence that sustained attention is not sustained at all but fluctuates with theta rhythms and alpha/beta rhythms. This provides yet more evidence that the brain works by rhythmic switching between representations.
A Dynamic Interplay within the Frontoparietal Network Underlies Rhythmic Spatial Attention
Neuron, Volume 99, Issue 4, 22 August 2018, Pages 842-853.e8
Neural Mechanisms of Sustained Attention Are Rhythmic
Neuron, Volume 99, Issue 4, 22 August 2018, Pages 854-865.e5
An excellent Preview by Rufin VanRullen: Attention Cycles
For further reading:
Buschman, T.J. and Miller, E.K. (2009) Serial, covert, shifts of attention during visual search are reflected by the frontal eye fields and correlated with population oscillations. Neuron, 63: 386-396. View PDF »
Buschman,T.J. and Miller, E.K. (2010) Shifting the Spotlight of Attention: Evidence for Discrete Computations in Cognition. Frontiers in Human Neuroscience. 4(194): 1-9. View PDF »