Pinotsis, D.A., Loonis, R., Bastos, A. Miller, E.K, and Friston, K.J. “Bayesian Modelling of Induced Responses and Neuronal Rhythms” Brain Topogr (2016). doi:10.1007/s10548-016-0526-y
Abstract:
Neural rhythms or oscillations are ubiquitous in neuroimaging data. These spectral responses have been linked to several cognitive processes; including working memory, attention, perceptual binding and neuronal coordination. In this paper, we show how Bayesian methods can be used to finesse the ill-posed problem of reconstructing—and explaining—oscillatory responses. We offer an overview of recent developments in this field, focusing on (i) the use of MEG data and Empirical Bayes to build hierarchical models for group analyses—and the identification of important sources of inter-subject variability and (ii) the construction of novel dynamic causal models of intralaminar recordings to explain layer-specific activity. We hope to show that electrophysiological measurements contain much more spatial information than is often thought: on the one hand, the dynamic causal modelling of non-invasive (low spatial resolution) electrophysiology can afford sub-millimetre (hyper-acute) resolution that is limited only by the (spatial) complexity of the underlying (dynamic causal) forward model. On the other hand, invasive microelectrode recordings (that penetrate different cortical layers) can reveal laminar-specific responses and elucidate hierarchical message passing and information processing within and between cortical regions at a macroscopic scale. In short, the careful and biophysically grounded modelling of sparse data enables one to characterise the neuronal architectures generating oscillations in a remarkable detail.
Morocos and Harvey reveal new depths in ongoing activity in parietal cortex in mice. Information about cues, behavioral choices, etc were not represented by single neurons in a winner-take fashion (the traditional view). Rather, different information is added to on-going patterns of activity that reflect the history of recent events. This could only be revealed via analysis of activity on single trials.
Morcos, Ari S., and Christopher D. Harvey. “History-dependent variability in population dynamics during evidence accumulation in cortex.” Nature Neuroscience (2016).
For another example of how single-trial analysis reveals much more than across-trial averaging, see:
Lunqvist, M., Rose, J., Herman, P, Brincat, S.L, Buschman, T.J., and Miller, E.K. (2016) Gamma and beta bursts underlie working memory. Neuron, published online March 17, 2016. View PDF »
Cavanagh et al show that characterizing the temporal receptive field of integration of individual PFC neurons from their resting activity (via autocorrelation) helps predict their coding for value. In short, taking into account the temporal dynamics of neuron spiking yields more information about their role in representing value than spike rates alone.
Cavanagh, Sean E., et al. “Autocorrelation structure at rest predicts value correlates of single neurons during reward-guided choice.” eLife 5 (2016): e18937.
Abstract:
As we learn about items in our environment, their neural representations become increasingly enriched with our acquired knowledge. But there is little understanding of how network dynamics and neural processing related to external information changes as it becomes laden with “internal” memories. We sampled spiking and local field potential activity simultaneously from multiple sites in the lateral prefrontal cortex (PFC) and the hippocampus (HPC)—regions critical for sensory associations—of monkeys performing an object paired-associate learning task. We found that in the PFC, evoked potentials to, and neural information about, external sensory stimulation decreased while induced beta-band (∼11–27 Hz) oscillatory power and synchrony associated with “top-down” or internal processing increased. By contrast, the HPC showed little evidence of learning-related changes in either spiking activity or network dynamics. The results suggest that during associative learning, PFC networks shift their resources from external to internal processing.
Brincat, S.L. and Miller, E.K (2016) Prefrontal networks shift from external to internal modes during learning Journal of Neuroscience. 36(37): 9739-9754, 2016 doi: 10.1523/JNEUROSCI.0274-16.2016. View PDF
Mixed selectivity in dopamine neurons:
Tian, Ju, et al. “Distributed and Mixed Information in Monosynaptic Inputs to Dopamine Neurons.” Neuron (2016).
For more on the importance of mixed selectivity see:
Fusi, S., Miller, E.K., and Rigotti, M. (2016) Why neurons mix: High dimensionality for higher cognition. Current Opinion in Neurobiology. 37:66-74 doi:10.1016/j.conb.2016.01.010. View PDF »
Rigotti, M., Barak, O., Warden, M.R., Wang, X., Daw, N.D., Miller, E.K., & Fusi, S. (2013) The importance of mixed selectivity in complex cognitive tasks. Nature, 497, 585-590, doi:10.1038/nature12160. View PDF »
Ott and Nieder show that stimulating dopamine D2 receptors enhancing working memory related activity in the prefrontal cortex.
Ott, Torben, and Andreas Nieder. “Dopamine D2 Receptors Enhance Population Dynamics in Primate Prefrontal Working Memory Circuits.”Cerebral Cortex (2016).
A very nice experiment from Matt Chafee et al (as usual). They show that neurons in the prefrontal cortex don’t have fixed properties. Instead, they show “mixed selectivity” that changes with behavioral context and is biased toward stimuli that inhibit prepotent responses. Sounds like cognitive control to me.
Blackman, Rachael K., et al. “Monkey prefrontal neurons reflect logical operations for cognitive control in a variant of the AX continuous performance task (AX-CPT).” The Journal of Neuroscience 36.14 (2016): 4067-4079.
The multidemand network is a set of frontoparietal areas in humans that are recruited for a wide range of cognitive-demanding tasks. Mitchell et al use FMRI connectivity analysis to identify a putative homolog in monkeys.
Ester et al addressed the dichotomy of source vs site in visual attention. The frontoparietal cortex has long been thought to be the “source” of top-down attention signals that enhance activity at “sites” in posterior (sensory) cortex that represent visual stimuli. They used fMRI, a roving searchlight analysis, and an inverted encoding model to show that stimulus representations are all over the cortex and enhanced by attention. This calls the dichotomy between source and site into question.
Ibos and Freedman show that spatial and feature-based attention independently modulate activity in area LIP and that they added together. This suggests a common function of gating task-relevant features, whether they are spatial or non-spatial.
Nice review and test of a hypothesis about the role of transient beta oscillations in cortical processing.
Sherman et al (2016) Neural mechanisms of transient neocortical beta rhythms: Converging evidence from humans, computational modeling, monkeys, and mice
For much of the history of modern neuroscience, it has been a assumed that the neuron is the functional unit of the brain. But now there is increasing evidence that ensembles of neurons, not individuals, are the functional units. One line of evidence is that many neurons in higher cortical areas have “mixed selectivity” , responses to diverse combinations of variables; they don’t signal one “message”. Thus, their activity only makes sense when simultaneously considering the activity of other neurons. In fact, we (Rigotti et al., 2013; Fusi et al., 2016) have shown that mixed selectivity gives the brain the computational horsepower needed for complex behavior.
In this paper, Dehaqani et al show that simultaneously recorded prefrontal cortex neurons have high-dimensional, mixed-selectivity, representations and convey more information as a population than even individuals. This was especially true for parts of visual space that were weakly encoded by single neurons. Less-informative neurons were recruited into ensemble to fully encode visual space.
Prefrontal neurons expand their representation of space by increase in dimensionality and decrease in noise correlation. Mohammad-Reza Dehaqani, Abdol-Hossein Vahabie, Mohammadbagher Parsa, Behrad Noudoost, Alireza Soltani
doi: http://dx.doi.org/10.1101/065581
Further reading:
Fusi, S., Miller, E.K., and Rigotti, M. (2016) Why neurons mix: High dimensionality for higher cognition. Current Opinion in Neurobiology. 37:66-74 doi:10.1016/j.conb.2016.01.010. View PDF »
Rigotti, M., Barak, O., Warden, M.R., Wang, X., Daw, N.D., Miller, E.K., & Fusi, S. (2013) The importance of mixed selectivity in complex cognitive tasks. Nature, 497, 585-590, doi:10.1038/nature12160. View PDF »
Yuste, Rafael. “From the neuron doctrine to neural networks.” Nature Reviews Neuroscience 16.8 (2015): 487-497.
Woolgar et al provide a meta-analysis of experiments using multivoxel pattern analysis in FMRI. They show that cortical areas traditionally though to be visual, auditory or motor, primarily (though not exclusively) code visual, auditory, and motor information. However, the frontoparietal cortex is hypothesized to a multiple-demand network and it shows domain generality, coding multisensory and rule information.
Woolgar, Alexandra, Jade Jackson, and John Duncan. “Coding of visual, auditory, rule, and response information in the brain: 10 years of multivoxel pattern analysis.” Journal of cognitive neuroscience (2016).
LIP has been the area for studying motion direction discrimination as model of decision-making. In this paper, Katz et al show that deactivation of LIP has little effect on that model task. Deactivating an upstream area, MT, where decision signals are weaker, however, caused a big deficit.
Dissociated functional significance of decision-related activity in the primate dorsal stream. Leor N. Katz, Jacob L. Yates, Jonathan W. Pillow & Alexander C. Huk Nature.
Sure, this is a cautionary tale of correlates does not equal causation. But it is important not to over-interpret the results of lesions/deactivations. They identify *bottlenecks* in neural processing, not contributions. Just because there is no effect of deactivation doesn’t mean that a given area doesn’t contribute. MT could be providing the raw materials that a number of downstream areas, including LIP, use for decision-making. This doesn’t mean that LIP doesn’t contribute to decisions, it just means that it is not the only area that contributes.
This is in line with recent work showing that neural processing is more distributed than previously thought. For example, see:
Siegel, M., Buschman, T.J., and Miller, E.K. (2015) Cortical information flow during flexible sensorimotor decisions. Science. 19 June 2015: 1352-1355. View PDF »
An excellent, comprehensive review of the neurobiology of decision-making by David Freedman and John Asaad.
Neuronal Mechanisms of Visual Categorization: An Abstract View on Decision Making
David J. Freedman and John A. Assad, Annual Review of Neuroscience, 2016
DOI: 10.1146/annurev-neuro-071714-033919
This study shows the role of alpha and beta oscillations in the prefrontal cortex and frontal eye fields in a classic test of cognitive control: anti-saccades. It also shows how these oscillatory patterns develop with adulthood.
Hwang, Kai, et al. “Frontal preparatory neural oscillations associated with cognitive control: A developmental study comparing young adults and adolescents.” NeuroImage (2016).
Miller Lab Alumnus Andreas Nieder tells you everything you need to know about the brain substrates of the sense of number:
Nieder, Andreas. “The neuronal code for number.” Nature Reviews Neuroscience (2016).
Nice review of past work on the neurobiology of working memory and capacity limits:
Constantinidis, Christos, and Torkel Klingberg. “The neuroscience of working memory capacity and training.” Nature Reviews Neuroscience (2016).
Although there is a caveat: More recent work suggests that the substrate of working memory is *not* sustained spiking activity. That is an artifact of cross-trial averaging. “Delay activity” is more sparse and bursty on single trials. This suggests a different memory substrate.
See:
Lunqvist, M., Rose, J., Herman, P, Brincat, S.L, Buschman, T.J., and Miller, E.K. (2016) Gamma and beta bursts underlie working memory. Neuron, published online March 17, 2016. View PDF
Stokes, Mark G. “‘Activity-silent’working memory in prefrontal cortex: a dynamic coding framework.” Trends in cognitive sciences 19.7 (2015): 394-405.
Stokes and Spaak review our recent work on single-trial analysis of working memory “delay” activity. This showed that the classic profile of sustained activity as the memory substrate is an artifact of averaging across trials. The assumption is that averaging cancels out noise. Instead, it may be covering up important details of the dynamics of neural activity.
Read more here:
The Importance of Single-Trial Analyses in Cognitive Neuroscience
Mark Stokes and Eelke Spaak
Trends in Cognitive Sciences
DOI: http://dx.doi.org/10.1016/j.tics.2016.05.008
The original paper:
Lunqvist, M., Rose, J., Herman, P, Brincat, S.L, Buschman, T.J., and Miller, E.K. (2016) Gamma and beta bursts underlie working memory. Neuron, published online March 17, 2016. View PDF »
Nice paper showing that different task demands in different task stages engage different oscillatory bands in the prefrontal cortex.
Wimmer, Klaus, et al. “Transitions between Multiband Oscillatory Patterns Characterize Memory-Guided Perceptual Decisions in Prefrontal Circuits.”The Journal of Neuroscience 36.2 (2016): 489-505.
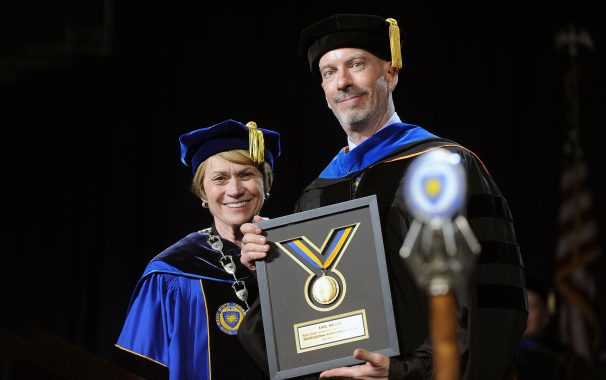
Earl Miller receives the Kent State University Professional Achievement Award from KSU President Beverly Warren.
And delivers the Commencement Address:
Free access to our new paper:
Lundqvist, M., Rose, J., Herman, P., Brincat, S. L., Buschman, T. J., & Miller, E. K. (2016). Gamma and Beta Bursts Underlie Working Memory. Neuron.
Valid until May 26, 2016
Tsutsui et al shows how the prefrontal cortex integrates rule and category information for a behavioral decision.
Tsutsui, Ken-Ichiro, et al. “Representation of Functional Category in the Monkey Prefrontal Cortex and Its Rule-Dependent Use for Behavioral Selection.” The Journal of Neuroscience 36.10 (2016): 3038-3048.
Michael Halassa, Guoping Feng, and colleagues show that a genetic deficit found in patients with ADHD produces (in mice) deficit in the thalamic reticular nucleus. This adds to Halassa’s recent work (also in Nature) suggesting that attention is focused when the prefrontal cortex acts on sensory cortex via the thalamus. It adds a link to potential path to treatment. Cool.
Thalamic reticular impairment underlies attention deficit in Ptchd1Y/− mice
Michael F. Wells, Ralf D. Wimmer, L. Ian Schmitt, Guoping Feng & Michael M. Halassa
Sustained activity has long been thought to be the neural substrate of working memory. But the evidence is based on averaging neural activity across trials. A closer examination reveals that something more complex is happening and supports a very different model of working memory.
Gamma and Beta Bursts Underlie Working Memory
Mikael Lundqvist, Jonas Rose, Pawel Herman, Scott L. Brincat, Timothy J. Buschman, Earl K. Miller
Neuron, published online March 17, 2016
Summary
Working memory is thought to result from sustained neuron spiking. However, computational models suggest complex dynamics with discrete oscillatory bursts. We analyzed local field potential (LFP) and spiking from the prefrontal cortex (PFC) of monkeys performing a working memory task. There were brief bursts of narrow-band gamma oscillations (45–100 Hz), varied in time and frequency, accompanying encoding and re-activation of sensory information. They appeared at a minority of recording sites associated with spiking reflecting the to-be-remembered items. Beta oscillations (20–35 Hz) also occurred in brief, variable bursts but reflected a default state interrupted by encoding and decoding. Only activity of neurons reflecting encoding/decoding correlated with changes in gamma burst rate. Thus, gamma bursts could gate access to, and prevent sensory interference with, working memory. This supports the hypothesis that working memory is manifested by discrete oscillatory dynamics and spiking, not sustained activity.